Tasks of Kubeflow – Introduction to Pipelines and Kubeflow
An input-driven job executes a component, called Task. It is a component template instantiation. A pipeline consists of jobs that may or may not share data. One pipeline component can instantiate numerous jobs. Using loops, conditions, and exit handlers, tasks may be generated and run dynamically. Because tasks represent component runtime execution, you may configure environment variables, hardware resource requirements, and other task-level parameters.
There are three types of task dependencies:
- Independent tasks: Depending on the circumstances, tasks may or may not be interdependent. If neither job depends on the results of the other, and vice versa, then we say that the two tasks are completely decoupled from one another. At pipeline runtime, two jobs can run simultaneously if they are independent.
- Implicitly dependent tasks: The creation of an implicit dependency between tasks occurs when the outcome of one job is the input to another. In this situation, the upper job will run before the latter, and the latter will get its results.
- Explicitly dependent tasks: It can be useful to control the order in which two activities run without sharing data between them. If this is the case, the subsequent job can be invoked by calling it. (.after() needs to be mentioned to create the sequence of execution between the tasks).
For this exercise, data is downloaded from Kaggle (link is provided below) and the dataset is listed under CC0:Public domain licenses (same dataset which we had used for AutoML for image data). For our exercise, we are considering images belonging to Cruise ships, ferry boat and Kayak (50 images are chosen randomly from each category).
https://www.kaggle.com/datasets/imsparsh/dockship-boat-type-classification
pipeline_automl bucket is created under us-centra1 (single region) and three folders containing images belonging to three categories are uploaded. CSV file is created and uploaded to same bucket containing the information regarding the full path of the image files, and the category they belong to as shown in Figure 6.1: (Refer to Vertex AI AutoML for Image data section of Chapter 6, AutoML Image, text and pre-built models to create the csv file):
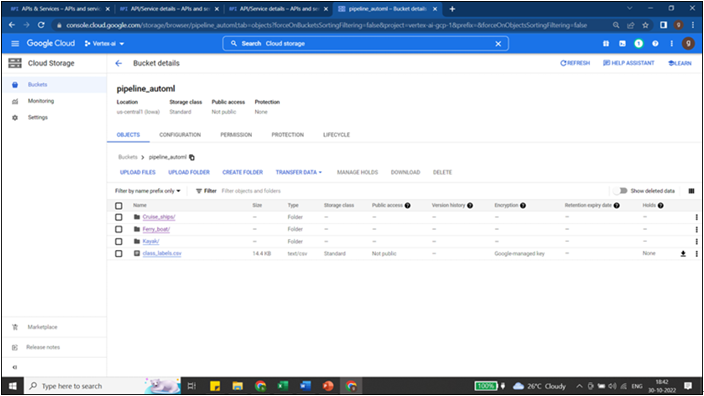
Figure 6.1: Data and csv uploaded to cloud storage bucket
In this chapter, we will create pipeline on Vertex AI component of GCP using Kubeflow SDK. In the pipeline we will use the AutoML component of GCP for model training.
You may also like
Archives
- September 2024
- August 2024
- July 2024
- June 2024
- May 2024
- April 2024
- March 2024
- February 2024
- January 2024
- December 2023
- November 2023
- September 2023
- August 2023
- June 2023
- May 2023
- April 2023
- February 2023
- January 2023
- November 2022
- October 2022
- September 2022
- August 2022
- June 2022
- April 2022
- March 2022
- February 2022
- January 2022
- December 2021
- November 2021
- October 2021
Calendar
M | T | W | T | F | S | S |
---|---|---|---|---|---|---|
1 | 2 | 3 | 4 | 5 | ||
6 | 7 | 8 | 9 | 10 | 11 | 12 |
13 | 14 | 15 | 16 | 17 | 18 | 19 |
20 | 21 | 22 | 23 | 24 | 25 | 26 |
27 | 28 | 29 | 30 | 31 |
Leave a Reply